Achieving process optimization in the field of bulk solids through smart solutions, AI, and deep learning
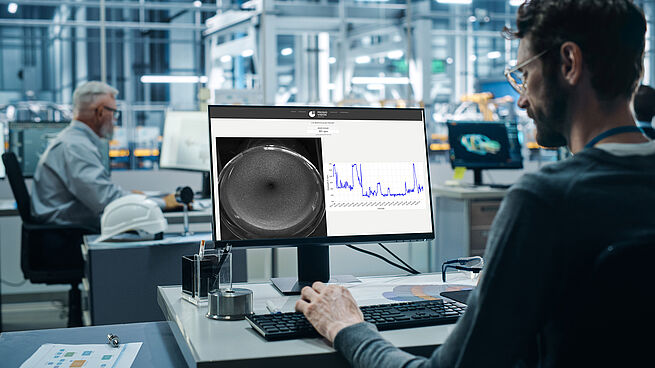
Digitalization of production processes with the aid of new technologies and intelligent networking of machinery and production lines is a real game changer for many companies.
Companies and products have to keep evolving in order to meet the requirements of the marketplace and of customers. With new technologies, processes can be optimized so that every aspect of production is completely transparent and consistently high quality is guaranteed at every step along the way. Innovative companies can gain a real competitive advantage by focusing on digitalization of both production and quality assurance.
The reason for this is that subprocesses need to optimally networked with each other in order to manufacture ever more complex products cost effectively. Assistance systems and smart data analysis are essential and make it possible to adjust production parameters to the actual operating situation.
Smart quality systems for autonomous analysis
In its digital solutions for process optimization, Eirich uses autonomous analysis tools, AI-based process assistance systems, and – as a brand new feature – product characterization with deep learning.
The granulate specialist VC1 is equipped with a special camera that continuously records, processes, and analyzes images from the product conveyor belt. In the process, the system identifies and calculates numerous parameters and product properties from the images. In the process, the assessment of the quality parameters can be performed by the person operating the system, or this can be handled by intelligent control algorithms. The data obtained in this way forms the basis for wide-ranging options for the control of complex production lines in order to optimize product quality and line efficiency.
QualiMaster VC2 features a universal camera system for automatic trend detection via AI-based image analysis, thus making it a universal instrument for quality assessment of materials of all types and consistencies. The smart quality system takes images of the product on the conveyor belt immediately downstream of the mixer. Based on the images, a decision is then taken whether the condition and consistency of the material matches the requirements, or whether undesirable trends are evident in terms of the material properties. In contrast to the VC1 system, VC2 does not measure any concrete values or parameters from the images, but instead uses deep learning – a sub-area of machine learning – to compare the actual results against reference images. One element that makes this possible is so-called neural networks, which are a form of self-learning algorithms. The more extensively the neural networks are trained on the relevant properties of a sample, the more precise the detection results become. As a result, quality control systems benefit from reliable and consistent classification of the investigated products. This makes it possible to analyze the materials in the process. The data obtained in this way can be used as a basis for intelligent closed-loop control processes that are capable of self-optimization.
Collecting and using process data the right way
Process digitalization starts with the measurement of important key parameters. This data must be recorded and analyzed systematically. In any production process, a wide range of data is always produced on a continuous basis. In the past, information was distributed on a “need-to-know” basis. This means that each piece of machinery or equipment is only sent exactly the data required for the relevant step in the process. As a result, interventions by the operator, for example from a control station, usually only act on individual sub-units. Consequently, to ensure efficient digitalization of production lines, the first step is to ensure that all necessary information is continuously collected and brought together in a single location. A number of solutions and platforms have been available for this for several years. However, since every production line is slightly different, each system needs to be individually tailored. This type of platform – usually on the basis of special databases – then forms the basis for the actual process optimization.
Every optimization task requires one or more target variables / key performance indicators (KPIs) or a target specification. Once again, these are also just as individual as each production process itself. While one line might attempt to maximize output, another might focus on avoiding rejects or faulty batches. Naturally, the objectives are usually more complex and generally comprise a combination of different KPIs and minimum requirements. Digitalization measures for process optimization are then used to obtain the optimum set of correcting variables for every conceivable operating point in the entire production process. Here, the optimization target in each case is to maximize all KPIs.
This is where intelligent sensor systems such as QualiMaster VC1 and VC2 come into play, and individual, isolated quality controls are no longer sufficient. An automatic closed-loop control system requires continuous information about product properties and qualities. Although it is not necessary to collect all conceivable types of information for complete, automated closed-loop process control, the key is to collect all the information that is necessary in order to transform a production site into a so-called dark factory.
The vision behind dark factories
Dark factories are a concept that is becoming increasingly important in modern manufacturing industries. In contrast to conventional factories, dark factories are highly automated and controlled by robots, as a result of which there is almost no need for human activities. However, the aspect that gives dark factories their name is that they can operate virtually without lighting, as all processes and machines are equipped with advanced sensors and artificial intelligence to enable precise and efficient production around the clock. This concept promises not only a significant increase in production speed and quality, but also a reduction in environmental burdens since it can help deliver energy savings and reduce the amounts of waste that are generated. Dark factories are a fascinating evolution of the manufacturing industry – one that could potentially play a major role in shaping the future of production technology.
Outlook: the future is now
Right now, the concept of dark factories may still sound like a far off vision of the future, as there are currently no real examples of this in the process industry. However, it is well worth taking a look at other areas in the value chain. In the final assembly of high-tech products with the aid of robots, dark factories are already a reality – for example at Philips in the Netherlands and FANUC in Japan. Apart from when maintenance or servicing work needs to be carried out, the lights stay out in the production halls. However, these sectors of industry employ quite different approaches when it comes to continuous quality monitoring, and the levels of standardization and automation are considerably higher. Nonetheless, these considerations should not put off companies from the process industry – quite the opposite, in fact. The vision of a production process that continuously delivers optimized results is a real possibility. The paths have already been highlighted – now we just have to follow them.
Author: Dr. Thomas Plankenbühler, CEO of prosio vision GmbH
The joint venture between Eirich and prosio engineering offers intelligent closed-loop control solutions for the bulk solids industry and is the AI center of the Eirich Group.